In today's data-driven world, the ability to effectively leverage GenAI, taxonomy, and ontology is no longer a luxury, but a necessity for organizations seeking clarity and a competitive edge.
Not long ago, “data was king.” Companies young and old raced to gather as much of it as possible, treating data as the ultimate competitive advantage. But fast-forward to today, and the landscape has changed: data is no longer scarce or expensive to generate. It’s cheap, everywhere, and in many cases, data overload and fatigue have become the new reality.
The challenge now? Making sense and extracting value from an over-abundance of data.
Taming Data Chaos with Taxonomy and Ontology Management
Organizations are overwhelmed by vast volumes of semi-structured and unstructured data; emails, internal wikis, websites, chat transcripts, old-fashioned documents, and customer feedback logs. In most cases, more information is generated than can be accurately processed. Finding the right piece of data isn’t just like finding a needle in a haystack, it’s more like searching for one specific needle in a haystack made entirely of needles.
We’ve all experienced it before. Whether it’s trying to find that one important line in a document, or searching your company’s knowledge base for a critical answer only to retrieve irrelevant and ambiguous results that slow you down and camouflage the exact piece of information needed. The frustration isn’t about having too much information, the challenge is the lack of a clear path to the right information when you need it now.
AI is often heralded as the solution to information overload. But the reality is, AI, especially large language models (LLMs), is only as reliable as the structure behind the data it draws from. Without a clear semantic foundation, even the most advanced systems can misclassify content, surface irrelevant results, or generate entirely inaccurate responses.
Taxonomies and ontologies are the missing components of the equation – the unsung heroes behind AI-powered insights, and they’re central to Taxonomy & Ontology Management Systems (TOMs) like Synaptica Graphite. With a TOMs-based AI approach, data is systematically classified, tagged, and governed, enabling LLMs to generate responses that are grounded in predefined logic rules and reason within the boundaries of the knowledge domain.
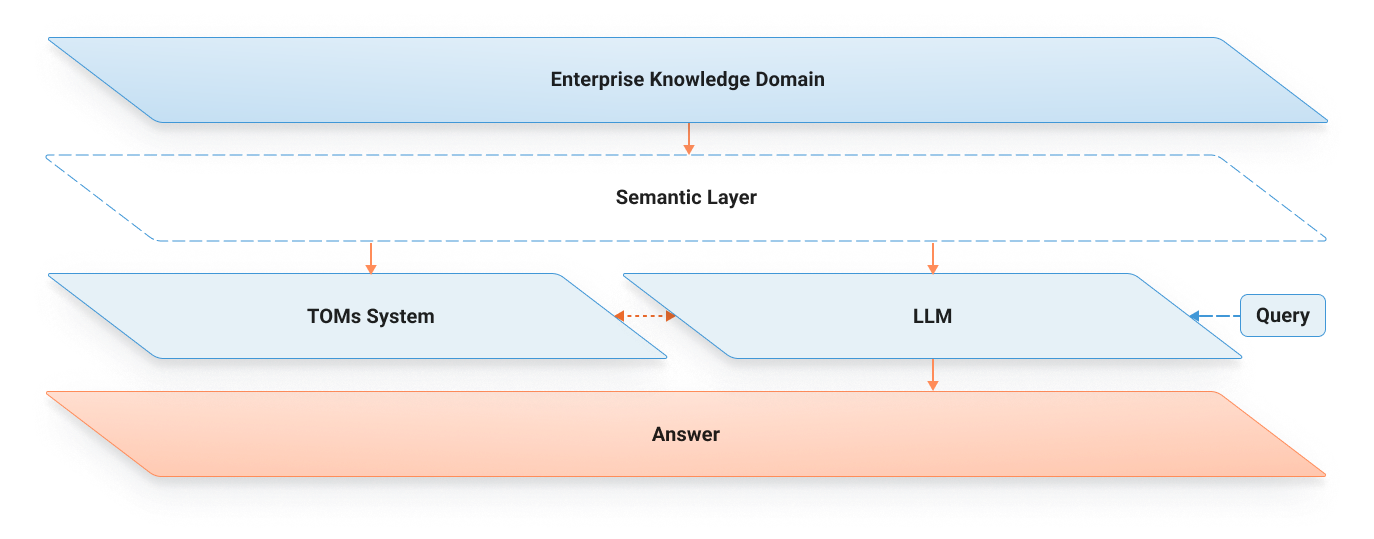
The Challenge of Unstructured Data: Similarity vs Meaning
Around 80% of enterprise data is unstructured. We’re talking about emails, policy documents, internal reports, and customer reviews, the kind of data that’s messy, diverse, and full of nuance. But it’s not just text: images, audio recordings, scanned PDFs, videos, and even sensor data all fall into the unstructured bucket. Think of compliance teams sorting through recorded customer calls, healthcare providers analyzing diagnostic images, or manufacturers interpreting IoT sensor logs.
This type of data doesn’t fit neatly into relational databases (like rows and columns). The meaning isn’t easily machine-readable without additional processing (e.g., natural language understanding, tagging). Even though they look structured to humans (organized in paragraphs, bullets, etc.), machines still see them as free text blobs unless they’re transformed into a structured, machine-readable format.
Harnessing Enterprise Taxonomy as a Bulwark Against AI Hallucinations
All the data holds immense value, but without proper organization, (or extremely specific prompts) it’s just noise. AI promises to handle it all. And yes, machine learning and LLMs excel at pattern recognition and trend spotting. But there’s a critical catch: these models work probabilistically. Their outputs are based on statistical likelihoods, not ground truths. That opens the door to: missed signals, misclassifications and AI “hallucinations,” - those moments when AI confidently gets things completely wrong.
For instance: an insurance company used AI to sift through customer complaints about "policy cancellations. But instead of surfacing only relevant cases, the system kept pulling in reports about "policy amendments" and "monthly statement closures." The AI saw similarity, but not meaning. Without a guiding framework, AI can only infer based on similarity and statistical probability. And for customers in regulated industries, such as finance and healthcare, that type of inference is not good enough.
What Taxonomies and Ontologies Do
Taxonomies and ontologies bring structure and semantic meaning to data. A taxonomy organizes concepts hierarchically, defining categories, synonyms, and preferred terms. An ontology goes further, mapping relationships, attributes, and rules to capture how those concepts connect and behave in context. Think of a taxonomy as the skeleton that holds the system together, and an ontology as the wiring that defines how everything interacts.
This is the core functionality of Synaptica Graphite, a no-code taxonomy & ontology management system (“TOMs”) that lets teams design, manage, and evolve rich taxonomies and ontologies based on Semantic Web standards and RDF modeling.
The advantages of a TOMs based AI approach are indisputable. With a taxonomy and ontology management system in place, an LLM classifier can disambiguate terms. For example, distinguishing between Jaguar the animal and Jaguar the car brand. That’s because the enterprise taxonomy defines the terms as separate entities, each with its own context, synonyms, and relationships.
Semantic Tagging Enhances GenAI Precision
Additionally, taxonomies and ontologies support semantic tagging by linking content to defined concepts and relationships. For instance: imagine a company analyzing customer reviews. The word "Apple" appears throughout, sometimes referring to the tech giant, other times to the fruit or colour in product descriptions. Without rules-based tagging, the AI might conflate both concepts together. But, with a taxonomy that maps Apple Inc. under “Technology Companies” and apple (fruit) under “Produce,” the classifier can tag each instance accurately and consistently.
TOMs build transparent, auditable processes, crucial for industries where compliance is mandatory. For example, a global pharmaceutical company uses a TOMs to classify and track clinical trial documentation. Each term whether “adverse reaction,” “protocol deviation,” or “informed consent” is linked to a specific definition in the ontology, with defined tagging rules. So when regulators audit the system, the company can demonstrate exactly how documents were categorized, what rules were applied, and how classification decisions were made. This level of traceability helps organizations meet regulatory requirements and gives internal teams confidence in the consistency of AI-assisted outputs.
TOMs in Action, Enhancing AI’s Strengths
This is where taxonomies, ontologies, and classifiers, combined with AI/LLMs, truly raise the bar for delivering accurate, actionable, and explainable outputs.
Synaptica Graphite and Squirro’s AI platform work in tandem to connect structure with intelligence. Graphite provides the semantic framework, an evolving taxonomy or ontology that reflects your domain knowledge. It defines terms, maps relationships, and applies consistent tagging rules. In doing so, it gives AI a shared understanding of context and meaning, enabling deterministic outputs that are relevant and explainable.
Squirro’s AI Classifier builds on this foundation, applying that semantic layer to tag and enrich your data, whether it is clean, unstructured, or somewhere in between. The result: high-precision classification and recall that cuts through noise and surfaces relevant, disambiguated content. It can not only classify and tag known concepts in your TOM system, but identify and extract new concepts to feed back into the taxonomy/ontology enriching the knowledge graph.
When integrated with an enterprise knowledge graph, taxonomies and ontologies do more than classify and tag, they become the structural backbone of enterprise knowledge. Concepts defined in the taxonomy and ontology software form the nodes and relationships within the graph (a.k.a. “edges”), enable LLMs to reason across connected ideas, trace provenance, and deliver richer, more contextualized insights. This fusion of semantic structure and graph intelligence transforms disconnected data into an interconnected, navigable source of truth.
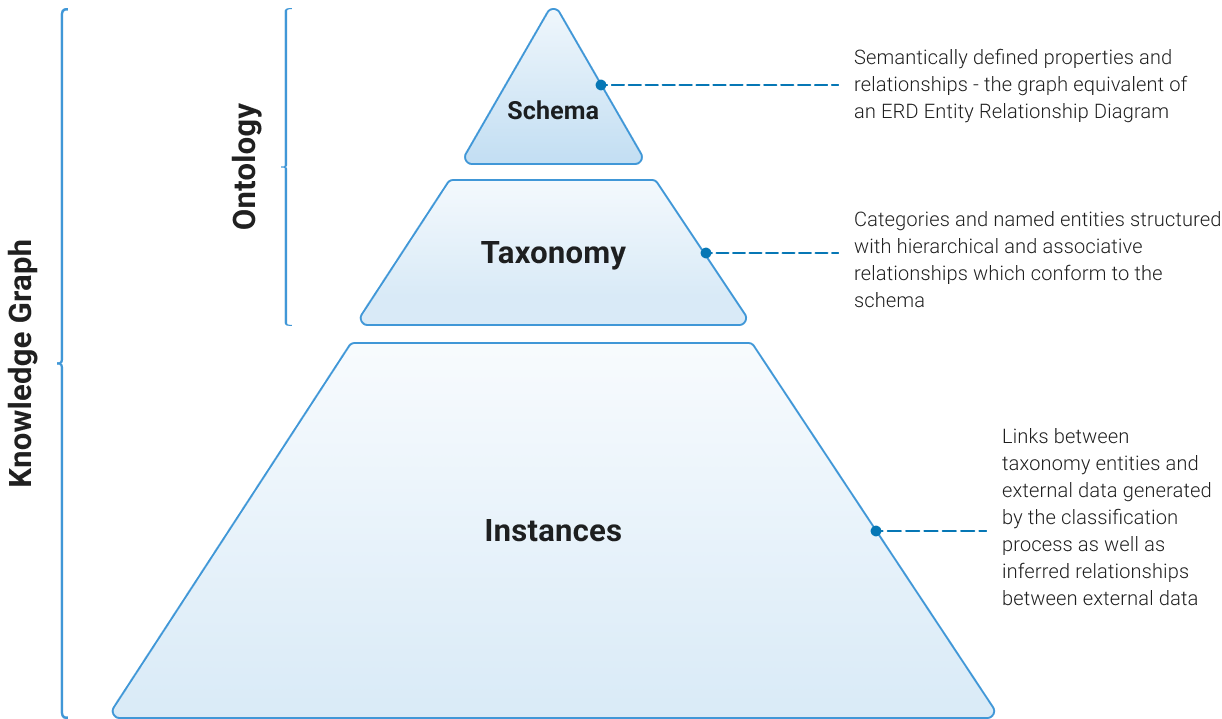
The strongest systems combine both approaches. Probabilistic AI brings speed, scale, and inference. Taxonomies and ontologies bring structure, control and relationship enabled reasoning. Together, they strike the balance between intelligence and accountability. That’s the strength of the Squirro + Synaptica approach: an AI system grounded in enterprise knowledge, capable of scaling without sacrificing precision.
A TOM-based approach to AI isn’t just an enhancement, it’s a strategic shift that makes intelligent systems truly reliable. By pairing AI with well-structured taxonomies and ontologies, organizations gain more than automation; they gain relevance, consistency, and control. Rather than relying on pattern recognition alone, AI becomes grounded in domain-specific logic, able to disambiguate, reason, and explain its outputs.
In a world where data is abundant but meaning is rare, taxonomies & ontologies provide the underlying structure AI needs to deliver trustworthy, context-aware results.
Download the Synaptica Guide and discover how you super charge your enterprise knowledge: https://squirro.com/kmworld-synaptica-guide
Access our in-depth white paper on "Advancing GenAI Beyond RAG "now and discover how the Squirro Enterprise GenAI Platform propels your enterprise AI strategy forward: https://squirro.com/advancing-genai-beyond-rag